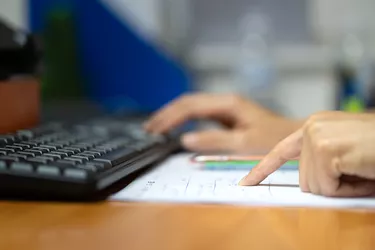
When trying to measure a quality of a population, it's often impractical or impossible to survey or measure the entire population. When basing conclusions on measuring a sample of the population, it's important to understand concepts like the sampling error and relative standard error or percent standard error. These values help convey the likely accuracy of the statistics.
Sources of Error in Statistics
Video of the Day
The field of statistics concerns measuring qualities about populations. A population is an entire set of units (people, marbles, fish) that generally have many qualities that could be measured (height/education level/opinions on a law; color/size/pattern; species/weight/sex). A sample is a subset of the population selected for measurement. When speaking about a quality of an entire population, this is called a parameter and represents the real prevalence or value of that quality in the population.
Video of the Day
When speaking about a quality in a sample, this is called a statistic and is an approximation of the real prevalence or value in the population. In other words, a statistic is an approximation of a parameter. Sampling error and percent standard error (also known as relative standard error, or RSE) are two ways of measuring and explaining how good that approximation probably is.
Errors in Statistics
Types of errors and ways of measuring and expressing them are major concerns in statistics because a statistic is not very useful if it's likely to be wrong, so it's important to be able to find ways to measure and express that wrongness.
For example, if you're trying to determine what percentage of college freshmen drink alcohol, you might put a team of interviewers on various college campuses that would randomly stop students they see and ask if they were freshmen and if they drank alcohol. The population would be all college freshmen in the United States, and the sample would be those that responded in the interviews. In this example, 5 percent of your sample reported drinking alcohol. However, since most college freshmen are under the age of 21 and it is illegal to drink alcohol at that age in the U.S., there's a high likelihood of respondents lying, especially when talking in person to an interviewer.
Additional Causes for Errors
Since it's expensive to send interviewers to a lot of college campuses and the number of people a single interviewer can talk to is limited, it's likely the study either did not involve a lot of campuses (or only involved ones close by), the study did not involve a lot of interviews or both.
The wording of the question may have been ambiguous, e.g. whether the interviewee drank regularly or had ever had alcohol by that point in their lives, such as in religious ceremonies. The interviewers may also have unconsciously targeted interviewees based on appearance or other characteristics, such as being more likely to approach white students or male-presenting students or those who appeared younger. The interviewees themselves may have been more or less likely to give an accurate response based on the characteristics of the interviewer, such as their race, gender or age and whether they appeared to be an authority figure.
The study may also have been less likely to reach certain populations, such as freshmen living off-campus or those taking mostly online courses. Between all of these factors, a study like this would likely not be very accurate.
Statistics and Government Studies
Many U.S. government agencies conduct statistical studies in relevant areas and provide explainers of their methodology and general education on statistical concepts as part of their public outreach. The National Oceanic and Atmospheric Administration (NOAA) and HIV.gov are two such sources. Scientists, government agencies and conscientious journalists are usually careful, precise and accessible in their reporting on statistics.
The NOAA explainer enumerates some types of statistical errors. The difference between sampling errors and non-sampling errors is one important distinction. Recalling that a sample is a portion of the population, a number of issues can arise. It's important for a sample to be representative of the population, or for the factors that make it less representative to be accounted for.
Sampling Errors and Representation
For example, in our survey of college freshmen, if the sample included 75 male-presenting interviewees and 25 female-presenting interviewees and did not verify gender while the population at large included 45 percent cis women, 45 percent cis men, and a total of 10 percent of other gender identities, there's a high likelihood that the sample was not representative of the population.
There are ways to adjust for known issues with how representative a sample is, such as weighting. However, one of the most straightforward ways of making a sample more representative is to increase the sample size. For example, a sample of 100 out of 330,000,000 (the approximate population of the US in 2021) is likely to be less representative than a sample of 100 out of a population of 1,000.
Sampling error describes and measures a specific category of errors, while percent standard error or relative standard error (RSE) are ways of measuring the precision and accuracy of statistics measured from a sample.
Sampling Error in Studies
Confusingly, "sampling error" does not refer to problems with selecting or working with a sample. Most such errors are actually referred to as "non-sampling errors," and the team at NOAA gives several examples. A coverage error occurs when the sample omits, duplicates or incorrectly includes items. In the example, fully-online students are likely to be omitted, students who misreport their class could be wrongly included and students who got interviewed twice would be duplicated. Measurement error is when the given response is incorrect, and in our example, this could occur because the interviewer went off-script, the question was ambiguously worded in the first place or when the students lie because the issue is controversial or sensitive.
Instead, "sampling error" refers to the difference between the average (mean) values measured in the sample and the actual mean in the population (the parameter). Of course, it's often impossible to know the exact sampling error because the actual mean is the object of study and is not known. However, if the parameter is known, the sampling error in the statistic can be measured, for example, as a way to determine how good the study methodology is and if it can be expanded.
When the actual parameter is known, then to calculate the sampling error, you simply subtract the observed from the actual, or the parameter minus the statistic. If you sample 20 marbles out of a jar of 100 black and white marbles and get 8 black marbles, you may get the statistic that 40 percent (8/20) marbles in the jar are black. However, if you count all the marbles and obtain the parameter that 50 (50 percent) of the marbles are black, then your sampling error would be 50 percent minus 40 percent, or 10 percent. The smaller the sampling error, the better.
Percent Standard Error
The percent standard error or relative standard error (RSE) is related to the standard deviation. The writers for Statistics How To explain that standard deviation and standard error are both measures of how spread out the values are. However, standard deviation is how spread out values in the population are while standard error is related to how spread out the values in your sample are.
Standard error can refer to a number of measures, such as the median versus the mean. The "standard deviation of the mean" refers to how different the average value in the sample is from the average value in the population, says the team at Statistics How To.
All of these calculations are often performed in software like Excel or programming languages like R. ExtendOffice explains how to calculate the standard error in Excel. You would need to use the following formula: Standard Error = STDEV(sample range)/SQRT(COUNT(sample range)). The University of Sheffield provides a textbook on data analysis and statistics with R, but since the standard error is the standard deviation divided by the square root of the sample size, a straightforward way to write a standard error function in R would be std <- function(x) sd(x)/sqrt(length(x)).
Consider also: How is Statistics Used in Insurance?